Trustworthy Scientific Machine Learning
PI: Dr. Xi Peng
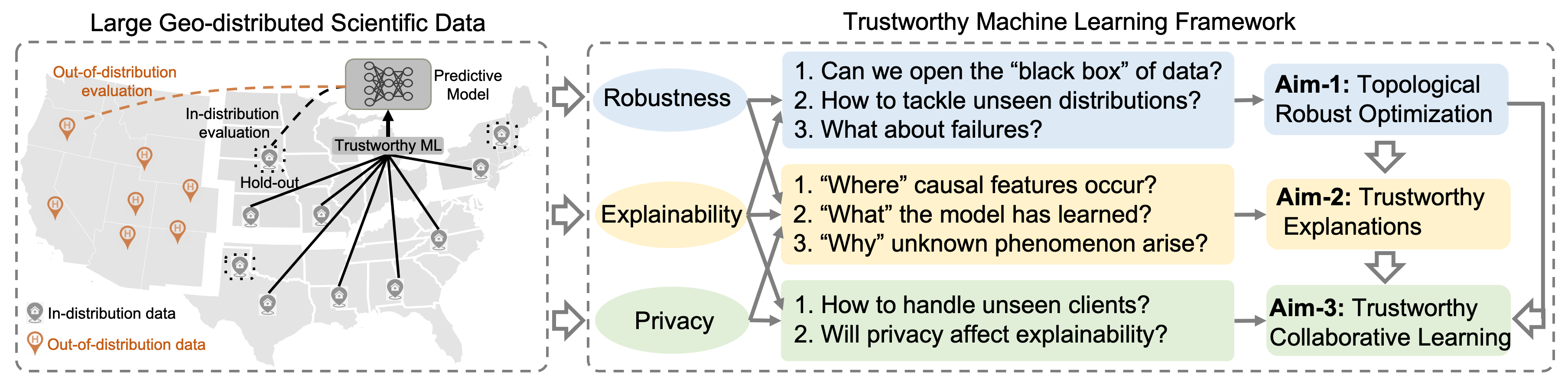
Trustworthy machine learning for geo-distributed scientific data analytics.
Description: This project aims to develop a trustworthy optimization toolbox for geo-distributed scientific data analytics, addressing gaps in AI/ML practices where models trained on historical or regional data struggle with complex and evolving dynamics of phenomena like extreme weather events and climate change. The project pioneers optimization methods to enhance prediction robustness, explanation reliability, and scalable privacy protections, crucial for rare or unseen scenarios in safety-critical applications. It pursues three aims: bridging data topology and robust optimization, revolutionizing explainable machine learning for scientific discovery, and ensuring trustworthy collaborative learning. The project integrates these advancements into education, promoting diversity and inclusion in STEM through interdisciplinary outreach and curricula.
Outcomes:
- [ICML'24] Fengchun Qiao and Xi Peng. Ensemble Pruning for Out-of-distribution Generalization. In International Conference on Machine Learning, 2024. [PDF] [Code]
- [ICML'24] Mengmeng Ma, Tang Li, Xi Peng. Beyond Federation: Topology-aware Federated Learning for Generalization to Unseen Clients. In International Conference on Machine Learning, 2024. [PDF] [Code]
- [ICLR'23] Fengchun Qiao and Xi Peng. Topology-aware Robust Optimization for Out-of-Distribution Generalization. In Proceedings of the International Conference on Learning Representations, 2023. [PDF] [Code]
- [CVPR'23] Tang Li, Fengchun Qiao, Mengmeng Ma, Xi Peng. Are Data-driven Explanations Robust against Out-of-distribution Data? In Proceedings of the IEEE Conference on Computer Vision and Pattern Recognition, 2023. [PDF] [Code] [Video]
- [TPAMI'22] Xi Peng, Fengchun Qiao, Long Zhao. Out-of-Domain Generalization from a Single Source: An Uncertainty Quantification Approach. IEEE Transactions on Pattern Analysis and Machine Intelligence, 2022. [PDF]
- [CVPR'21] Fengchun Qiao and Xi Peng. Uncertainty-guided Model Generalization to Unseen Domains. In Proceedings of the IEEE Conference on Computer Vision and Pattern Recognition, 2021. [PDF] [Code] [Video]
- [CVPR'20] Fengchun Qiao, Long Zhao, and Xi Peng. Learning to learn single domain generalization. In Proceedings of the IEEE Conference on Computer Vision and Pattern Recognition, 2020. [PDF] [Code] [Video]
Double-correct Prediction in Sciences
PI: Dr. Xi Peng (Machine Learning)
Co-PI: Dr. Rudolf Eigenmann (HPC)

A trustworthy toolbox for double-correct predictive modeling in sciences.
Description: AI and ML have driven scientific advances in critical domains like climate change and extreme weather prediction, but challenges remain due to unpredictable data shifts and unseen variables. This project introduces a novel trustworthy toolbox prioritizing both prediction robustness and rationale validity, ensuring accurate outcomes are backed by scientifically grounded rationales, even with unforeseen data variations. The toolbox will be optimized for scalability on HPC and released as open-source software, benefiting researchers in Earth, Marine, and Environmental Sciences through accessible, generalizable workflows and AI-ready datasets.
Outcomes:
- [NeurIPS'24] Tang Li, Mengmeng Ma, Xi Peng. Beyond Accuracy: Ensuring Correct Predictions with Correct Rationales In Proceedings of Advances in Neural Information Processing Systems, 2024. [PDF] [Code]
- [ECCV'24 Strong Double Blind] Tang Li, Mengmeng Ma, Xi Peng. DEAL: Disentangle and Localize Concept-level Explanations for VLM. In European Conference on Computer Vision, 2024. [PDF] [Code]
Safe Learning-enable System
PI: Dr. Xi Peng (Machine Learning)
Co-PI: Dr. Weisong Shi (Autonomous Vehicle)
Co-PI: Dr. Chengmo Yang (Hardware)
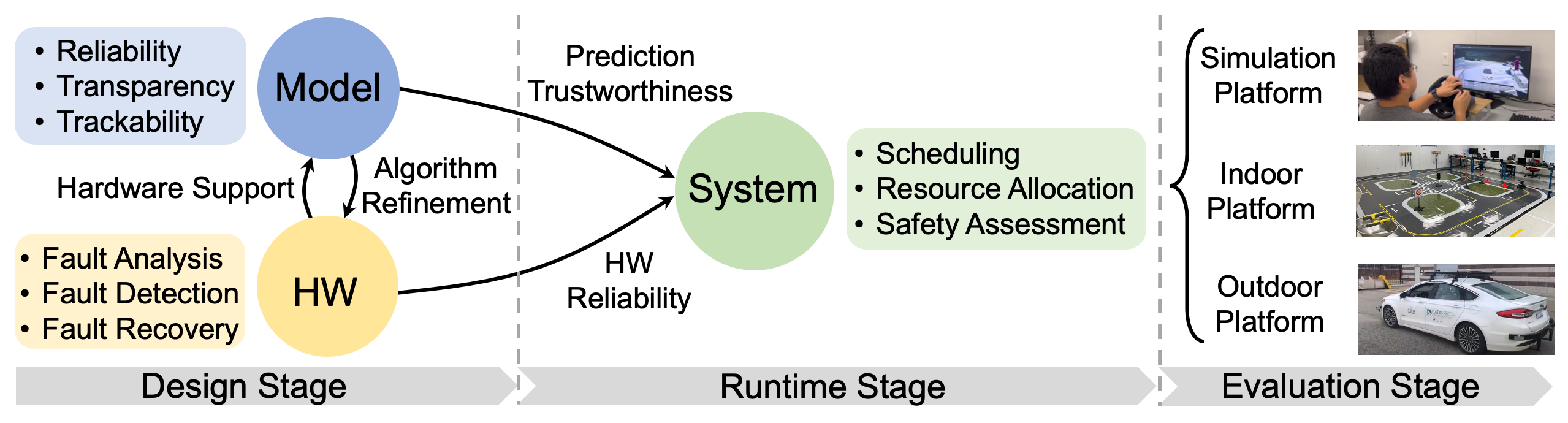
The proposed OSLA (Orchestrated Safe Learning for Autonomous driving) system.
Description: Machine Learning (ML) has transformed autonomous driving by enabling vehicles to perceive their environment with high precision, make real-time decisions, and operate without human intervention. However, unsafety may stem from the model—such as inappropriate extrapolation in unique scenarios—the hardware, which suffers from faults and errors, or the system, where the real-time operating system (RTOS) may not deliver decisions in time. Developing a safe learning-enabled system for autonomous vehicles (AVs) requires orchestrating the model, hardware, and system. This project focuses on cross-layer optimizations to achieve end-to-end safety by developing rational ML models with valid rationales, integrating hardware reliability into ML design to tolerate runtime faults, and designing an RTOS scheduler that ensures time predictability while considering model and hardware reliability. Implementing these advancements on real autonomous driving platforms will enhance AV safety, promote efficient transportation, and advance education and workforce development in AI and autonomous driving with a commitment to diversity and inclusion in STEM fields.
Outcomes:
- [NeurIPS'24] Tang Li, Mengmeng Ma, Xi Peng. Beyond Accuracy: Ensuring Correct Predictions with Correct Rationales In Proceedings of Advances in Neural Information Processing Systems, 2024. [PDF]
Robust & Explainable Seafloor AI
PI: Dr. Xi Peng (Machine Learning)
Co-PI: Dr. Arthur Trembanis (Marine Science)
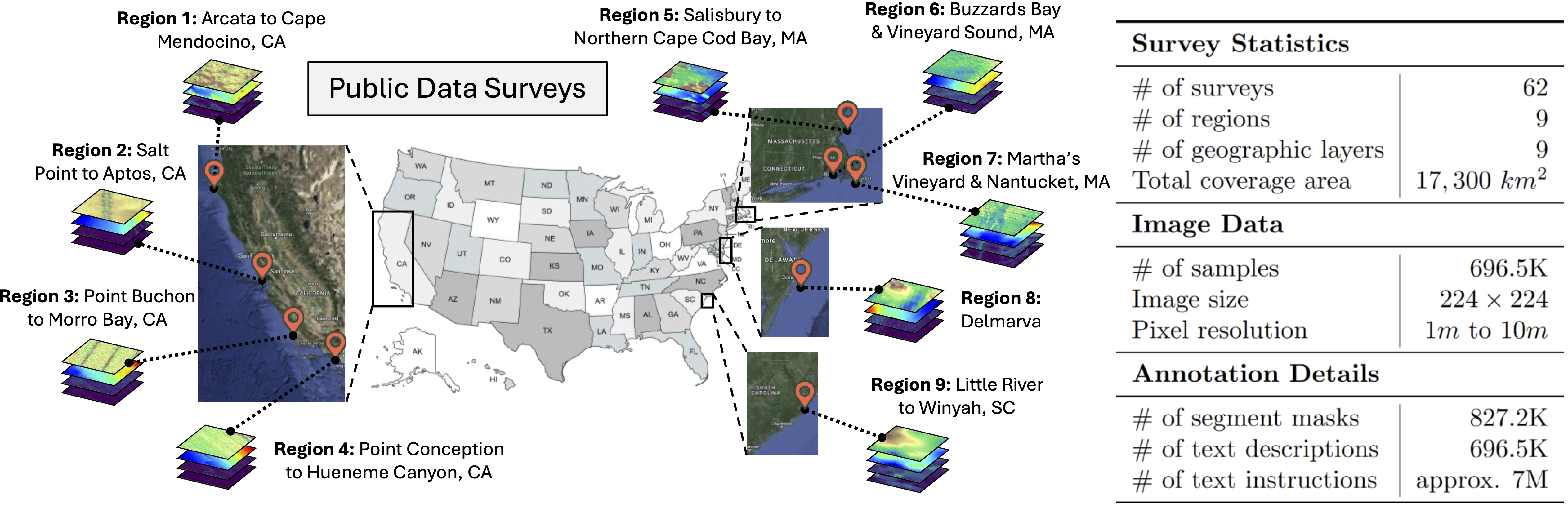
SeafloorAI, the first large AI-ready dataset for seafloor mapping using sonar imagery.
Description: Characterizing seafloor morphodynamics is essential for naval applications relying on extensive geoacoustic and environmental data over broad spatiotemporal scales. Understanding dynamics and uncertainty among numerous variables presents a significant out-of-distribution challenge, as AI/ML models often struggle with unseen distributions, leading to fragile predictions and unreliable explanations. To overcome these challenges, this project will develop new robust and explainable optimization methods using newly-curated seafloorAI datasets. The research outcomes—including an AI-ready multi-site seabed morphodynamic database, innovative trustworthy AI/ML optimization methods, and scalable implementations—will be linked to the Ocean Biogeographic Information System and Seabed 2030 to benefit a broad range of communities and stakeholders.
Outcomes:
- [NeurIPS'24] Kien X. Nguyen, Arthur Trembanis, Xi Peng. SeafloorAI: A Large-scale Vision-Language Dataset for Seafloor Geological Survey. In Proceedings of Advances in Neural Information Processing Systems Datasets and Benchmarks Track, 2024. [PDF] [Dataset]
- [CIKM'24] Kien X. Nguyen, Fengchun Qiao, Xi Peng. Adaptive Cascading Network for Continual Test-Time Adaptation. In Conference on Information and Knowledge Management, 2024. [PDF]
Safe AI for Prostate Cancer Diagnosis
PI: Dr. Xi Peng
Description: Advances in natural language processing can build on image processing breakthroughs to offer clinicians new AI tools against prostate cancer (PCa). Current AI for interpreting mp-MRI scans relies on visual encodings like lesion annotations but fails at translation in patient care because it doesn't use the standardized PI-RADS (Prostate Imaging Reporting and Data System) format accepted by clinicians. The expertise in PI-RADS reports offers a major resource for training AI to achieve clinical acceptance. This research addresses two gaps: (1) Data availability—public PCa data repositories lack PI-RADS reports, and (2) AI modeling—existing approaches can't integrate complex radiologist expertise expressed through language. We will test the hypothesis that PI-RADS reports can be made machine-readable and combined with visual data so that AI can be trained to interpret MRIs according to the reasoning processes of radiologists. University of Delaware researchers and Memorial Sloan Kettering radiologists will collaborate to develop datasets and tools for safe AI-assisted prostate MRI interpretation.
Outcomes:
- [CVPR'22] Mengmeng Ma, Jian Ren, Long Zhao, Davide Testuggine, Xi Peng. Are Multimodal Transformers Robust to Missing Modality? In Proceedings of the IEEE Conference on Computer Vision and Pattern Recognition, 2022. [PDF] [Video]
- [AAAI'21] Mengmeng Ma, Jian Ren, Long Zhao, Sergey Tulyakov, Cathy Wu, Xi Peng. Multimodal learning with severely missing modality. In Proceedings of the Association for the Advancement of Artificial Intelligence, 2020. [PDF] [Code] [Video]
Characterizing the Global Illicit Trade
Co-PI: Dr. Xi Peng (Machine Learning) with Dr. Julie Klinger (PI, Geo Science)
Description: The objective of this five-year project is to map and characterize the volume of illicitly-sourced materials in energy-critical minerals (ECM) supply chains. ECMs are essential to renewable, nuclear, and fossil energy generation and are included in the US governments list of 35 critical materials, yet their supply chains remain opaque and vulnerable to illicit activity. There are currently no global measurements of the licit-illicit composition of ECM trade flows or their evolution over time. To address the problem, this project seeks to map and model global ECM flows based on original research in several source, transit, and destination countries. The findings and tools developed under this study will improve discovery and traceability of illicitly sourced ECM, identify vulnerable points along several ECM supply chains, and generate predictive models of their dynamics in order to identify effective disruption strategies. The results will be informed by data drawn from open and proprietary datasets, as well as data gathered at national and subnational levels, and tested under extensive field research.
Outcomes:
- [ICLR'23] Fengchun Qiao and Xi Peng. Topology-aware Robust Optimization for Out-of-Distribution Generalization. In Proceedings of the International Conference on Learning Representations, 2023. [PDF] [Code]
- [ICCV'23] Qitong Wang, Long Zhao, Liangzhe Yuan, Ting Liu, Xi Peng. Learning from Semantic Alignment between Unpaired Multiviews for Egocentric Video Recognition. In Proceedings of the IEEE International Conference on Computer Vision, 2023. [PDF]
- [CVPR'22] Mengmeng Ma, Jian Ren, Long Zhao, Davide Testuggine, Xi Peng. Are Multimodal Transformers Robust to Missing Modality? In Proceedings of the IEEE Conference on Computer Vision and Pattern Recognition, 2022. [PDF] [Video]
- [TPAMI'22] Xi Peng, Fengchun Qiao, Long Zhao. Out-of-Domain Generalization from a Single Source: An Uncertainty Quantification Approach. IEEE Transactions on Pattern Analysis and Machine Intelligence, 2022. [PDF]
- [NeurIPSW'21 Best Paper Award] Tang Li, Jing Gao, Xi Peng. Deep Learning for Spatiotemporal Modeling of Urbanization. In Proceedings of Advances in Neural Information Processing Systems, Machine Learning for Public Health Workshop, 2021. [PDF] [Video] [Best Paper Award]